BLOG
Is the ChatGPT app safe? Discover hidden security flaws in ChatGPT’s Android app, real risks, and expert tips to protect your data—read before you install!
Discover how generative AI is changing the application security landscape and how you can turn it into an ally to solidify your security framework...
Artificial Intelligence is reshaping how we interact with technology, from virtual assistants to advanced search engines. However...
Featured blogs
Expert insights on navigating application security challenges
While SDLC ensures development efficiency & monitors time and budget constraints, SSDLC integrates security at each step.
"In an era where the scope and meaning of cyber hygiene are constantly shifting...
Artificial Intelligence is reshaping how we interact with technology, from virtual assistants to advanced search engines.
Explore our blogs
BLOG
API Security Testing Checklist for Developers
BLOG
OWASP MASTG Best Practices Checklist for Mobile ...
BLOG
Mastering MobSF: Everything You Need to Know ...
BLOG
How Safe is the ChatGPT Android App? An Appknox ...
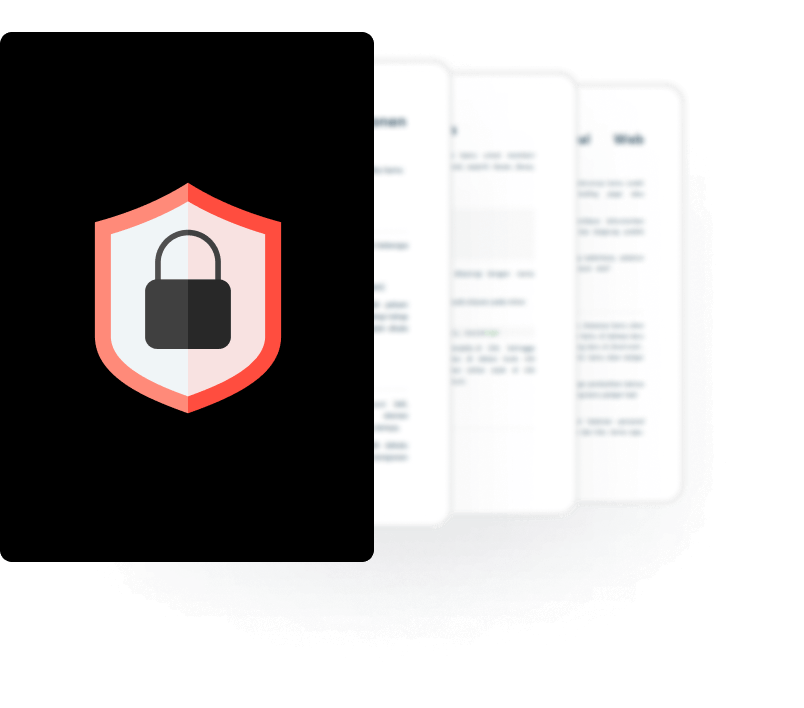